EN – Data Pipelines for Analytics
Data Pipelines for Analytics
An efficient data pipeline to achieve the expected results in advanced analytics actions.
Un pipeline de datos eficiente para conseguir los resultados esperados en las acciones de analítica avanzada.
The reality of Analytics Departments
The technical requirements of Analytics Departments change over time and so do the platforms used (SAS, Python, R and recently, Databricks). During all this time, none of these technologies has stopped being used and this generates a series of recurring problems: information silos, different data latencies, different data origins and quality, etc.
80% of Data Scientists’ time is dedicated to “fixing” the data: obtaining, cleaning, enriching, transforming, etc. This part of “Data Engineering” is crucial to have valid results.
And as always, “garbage in, garbage out”. If we don’t have the right data, the conclusions we draw from it won’t be right either, no matter how much we have used the best machine learning models.
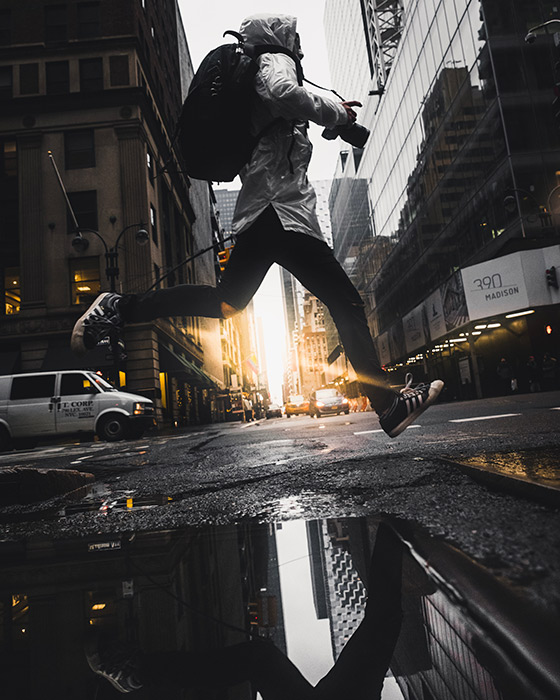
INITIATIVES
Benefits of the corporate data pipeline
- Measurable results in the short term. To do this we must take into account the following phases:
- Finding the right data.
- Cataloging the data to be used.
- Measuring its quality.
- Comply with privacy requirements.
- Reduced maintenance costs and greater flexibility when adding new data or modifying existing data.
- Simplicity when integrating data from different origins or with different latencies without “dying in the attempt”.
In corporate environments, we must equip ourselves with tools that allow us to industrialize these tasks and bring them to reality at reasonable costs. A long-term strategy is not possible without thinking that we must be resilient to technological changes, to changes in data models, to changes in programming languages or in where and how we store the data that we will later analyze.